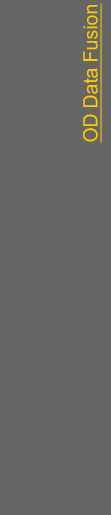 |
Methods
for estimating origin-destination (OD) trip matrices based on flow
counts on transport network links have been an established aspect
of transport modelling since the late 1980s and are widely applied.
Motivated by interests in maximising the exploitation of data increasingly
available from instrumented highways, as well ensuring that OD matrices
are estimated in the most appropriate way, the UK Highways Agency
(HA) commissioned this research project aimed at enhancing the development
of OD matrices. The project was undertaken by Hyder Consultancy and
its project partners, including a prominent role for Minnerva. The
study focused on the 'fusion' of count data with prior matrix data,
but the researched methodology was designed to support a wider range
of data sources, including from ANPR and GPS-based instruments
While some theoretical alternatives exist, current
matrix estimation methods may be seen as variations on a common
theme, which uses an 'objective function' to guide the adjustment
of a prior trip matrix to match information on input observed flows.
The translation from flows to matrix cell values involves information
on vehicle (or passenger) routeings, which is taken from network
modelling sources. The project, known as 'OD Data
Fusion', developed a generic simulation-based methodology for investigating
the choice of objective function, such as Maximum Likelihood, Maximum
Entropy, or Generalised Least Squares, and the use and settings
of weightings accorded to different sets of data to account for
their reliability. The methodology was studied using sets of synthetic
data for which a ‘true’ OD matrix was known, which is
never possible with real data. Real data was taken from two recent
HA network modelling projects, corresponding to 'small' and 'large'
scales to assess the methodology in relation to actual applications.
A feature of the methodology, which
was implemented using MATLAB software, was the integral consideration
of variability described by probability distribution functions (pdfs),
rather than simple mean values that are typically used. The study
explored different levels of variability on the results using assumptions
involving Poisson and Normal distributions, but part of the study
also analysed extensive sets of traffic count data and used Bayesian
Monte Carlo Markov Chain simulation modelling to implement a hierarchical
mixture model of pdfs to reflect more properly the distributions
observed in actual data. The methodology both exploited information
about the variability of input data (described using pdfs) and provided
equivalent output information on the variability for its outputs,
whether in terms of matrix cells or flows on links
Contact: Miles
Logie
|
|
 |